Embark on an intriguing journey through AP Stats Unit 7 MCQ Part C, where we unravel the captivating world of proportions and hypothesis testing. From understanding confidence intervals to conducting z-tests, this unit promises an adventure that will ignite your statistical curiosity.
Prepare to delve into the depths of statistical inference, where we’ll explore the intricacies of estimating population proportions and testing hypotheses about them. Along the way, we’ll uncover the secrets of comparing proportions and determining the optimal sample size for accurate estimations.
Statistical Inference for Proportions
Statistical inference for proportions involves making inferences about the population proportion based on a sample proportion. We use confidence intervals to estimate the true population proportion with a certain level of confidence.
Calculating a Confidence Interval for a Population Proportion
To calculate a confidence interval for a population proportion, we use the normal distribution and the sample proportion. The formula for the confidence interval is:
CI = p ± z
√(p(1-p)/n)
where:
- CI is the confidence interval
- p is the sample proportion
- z is the z-score corresponding to the desired confidence level
- n is the sample size
Margin of Error for a Confidence Interval
The margin of error (ME) is half the width of the confidence interval. It represents the maximum amount by which the sample proportion can differ from the true population proportion.
ME = z
√(p(1-p)/n)
Hypothesis Testing for Proportions: Ap Stats Unit 7 Mcq Part C
Hypothesis testing is a statistical method used to determine whether a claim about a population parameter is supported by the available evidence from a sample. In the case of proportions, we are interested in testing claims about the proportion of a population that possesses a certain characteristic.
When you’re tackling AP Stats Unit 7 MCQ Part C, you might find yourself needing to brush up on your vocabulary. For instance, if you’re unsure about the meaning of “confounding variable,” check out vocabulary unit 5 level e . It has helpful definitions and examples that can clear up any confusion.
Once you’ve got your vocabulary down, you’ll be well-equipped to conquer those AP Stats Unit 7 MCQ Part C questions.
The steps involved in hypothesis testing for a population proportion are as follows:
- State the null hypothesis (H0) and the alternative hypothesis (Ha).
- Set the level of significance (α).
- Calculate the test statistic.
- Determine the p-value.
- Make a decision.
Null Hypothesis and Alternative Hypothesis, Ap stats unit 7 mcq part c
The null hypothesis (H0) represents the claim that is being tested. It typically states that there is no significant difference between the population proportion and a specified value.
The alternative hypothesis (Ha) represents the claim that is being proposed as an alternative to the null hypothesis. It typically states that there is a significant difference between the population proportion and the specified value.
Example
Suppose we want to test the claim that the proportion of adults who own a smartphone is 0.80. We collect a sample of 200 adults and find that 160 of them own a smartphone.
We can conduct a hypothesis test using the z-test to determine whether the sample provides sufficient evidence to reject the claim that the population proportion is 0.80.
The steps involved in this hypothesis test are as follows:
- State the null hypothesis (H0): p = 0.80
- State the alternative hypothesis (Ha): p ≠ 0.80
- Set the level of significance (α): 0.05
- Calculate the test statistic: z = (0.8
- 0.80) / sqrt(0.80
- 0.20 / 200) = 0
- Determine the p-value: p = 2
P(Z< 0) = 1
- Make a decision: Since the p-value is greater than the level of significance, we fail to reject the null hypothesis. This means that we do not have sufficient evidence to conclude that the population proportion is different from 0.80.
Comparing Two Proportions
In statistical inference, comparing two population proportions is crucial for assessing the differences between two groups or populations. Two widely used methods for this comparison are the two-sample z-test and the chi-square test, each with its own assumptions and conditions.
Two-Sample z-Test
The two-sample z-test is appropriate when the sample sizes are large (n 1and n 2both greater than 30) and the populations are normally distributed or the sample sizes are large enough to invoke the Central Limit Theorem. The assumptions of the two-sample z-test include:
- Random and independent samples from each population.
- Sample sizes (n 1and n 2) greater than 30.
- Normally distributed populations or large enough sample sizes to invoke the Central Limit Theorem.
Chi-Square Test
The chi-square test is an alternative method for comparing two population proportions, particularly suitable when the sample sizes are small (less than 30) or the populations are not normally distributed. The assumptions of the chi-square test include:
- Random and independent samples from each population.
- Expected frequencies in each cell of the contingency table should be at least 5.
Example: Two-Sample z-Test
Suppose we want to compare the proportion of students who prefer online learning in two different schools. School A has 200 students, and 120 of them prefer online learning. School B has 300 students, and 150 of them prefer online learning.
Using the two-sample z-test, we can test the hypothesis that the proportion of students who prefer online learning is the same in both schools.
The null hypothesis is H 0: p 1= p 2, and the alternative hypothesis is H 1: p 1≠ p 2.
The sample proportions are: “` p 1= 120/200 = 0.6 p 2= 150/300 = 0.5 “`
The pooled proportion is: “` p = (120 + 150) / (200 + 300) = 0.55 “`
The standard error of the difference between the two proportions is: “` SE = sqrt(p*(1-p)*(1/n 1+ 1/n 2)) = sqrt(0.55*0.45*(1/200 + 1/300)) = 0.049 “`
The z-statistic is: “` z = (p 1– p 2) / SE = (0.6 – 0.5) / 0.049 = 2.04 “`
The p-value is: “` p-value = 2*P(Z > 2.04) = 2*0.0207 = 0.0414 “`
Since the p-value (0.0414) is less than the significance level (0.05), we reject the null hypothesis and conclude that the proportion of students who prefer online learning is not the same in the two schools.
Sample Size Determination for Proportions
Determining the appropriate sample size is crucial for accurate population proportion estimation. Several factors influence the sample size, including the desired margin of error, confidence level, and expected population proportion.
Formula for Sample Size Calculation
The sample size (n) required to estimate a population proportion (p) with a margin of error (e) and confidence level (1-α) is given by:
n = (Z^2
- p
- q) / e^2
where:
- Z is the z-score corresponding to the desired confidence level
- p is the expected population proportion
- q = 1 – p
Example
Suppose we want to estimate the proportion of students who own a laptop with a margin of error of 5% and a confidence level of 95%. Assuming no prior knowledge about the population proportion, we can use p = q = 0.5.
Using the formula, we get:
n = (1.96^2
- 0.5
- 0.5) / 0.05^2 = 384.16
Therefore, a sample size of 385 students would be required to achieve the desired accuracy and confidence level.
Question Bank
What is the margin of error in hypothesis testing?
The margin of error represents the amount of sampling error that is tolerated in a hypothesis test. It is calculated as half the width of the confidence interval.
How do I determine the sample size for estimating a population proportion?
The sample size is determined using a formula that considers the desired margin of error, confidence level, and estimated population proportion.
What is the difference between a null hypothesis and an alternative hypothesis?
In hypothesis testing, the null hypothesis represents the claim that there is no significant difference or effect, while the alternative hypothesis represents the claim that there is a significant difference or effect.
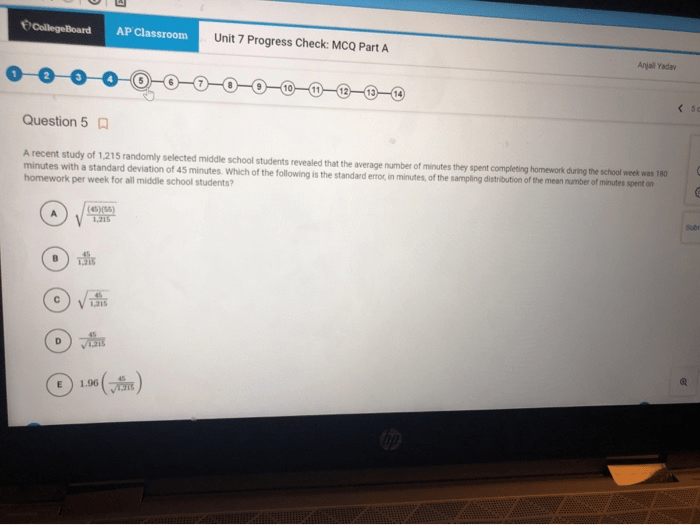